Data has gravity, and Snowflake and Databricks* proved this last week at their annual user summits—Snowflake’s in Las Vegas and Databricks’ in San Francisco. The two companies once served related but separate corners of the corporate-data market, but they’re now on a collision course to win the large and rapidly emerging AI/ML opportunity, with billions at stake.
Frank Slootman, the CEO of Snowflake, kicked off his keynote at the Snowflake conference by noting that “in order to have an AI strategy, you have to have a data strategy.” It’s a relevant comment as the ongoing debate around whether this next generation of AI productivity will be model-driven or data-driven continues. As use cases mature and AI developer tool stacks materialize, what’s becoming increasingly clear is ML models can only be as good as the underlying data that’s feeding them, and data is going to be a key differentiator.
In this vein, both Databricks and Snowflake are well-positioned to tackle AI as their respective products already serve as the backbone of many companies’ data strategies; enterprises hold vast amounts of valuable, and proprietary, first-party data that’s going to be critical in powering the next generation of intelligent, AI-driven applications.
Yet, access to data alone is not going to be enough, and companies adopting AI also need the right tools to support data retrieval, integration, and augmentation, which is already rapidly emerging with vector databases like Weaviate* and Pinecone, model-agents such as LangChain and LlamaIndex, and new prompting techniques like Retrieval Augmented Generation or RAG. All of these empower companies to combine the knowledge baked into model parameters with an external corpus of data.
The biggest takeaway from the two conferences, to us, was the theme of bringing models/compute closer to the troves of proprietary, enterprise data that already exists inside of Databricks and Snowflake. While we have long debated the end state of how enterprises will leverage AI in production—either through sending data directly to off-the-shelf, third-party model providers like OpenAI, Cohere, or Anthropic, or bringing models, both third-party and open- source, directly to the data—both Databricks and Snowflake have made it abundantly clear that data has gravity. And, despite the size, sophistication, and abstraction that off-the-shelf, third party models offer, enterprises want the ability to train, fine-tune, and run models directly on top of their proprietary, first-party data without compromising on performance, cost, and security and governance concerns.
While the announcements around Generative AI dominated the keynotes and breakout sessions at both companies’ user conference, we wanted to summarize a few other key observations that we believe are worth noting.
End-to-end platforms:
- A data platform is only valuable if it enables the translation of raw data into actionable intelligence. Over the last couple of years both Databricks and Snowflake have transitioned from being cloud-data “lakehouse” providers to horizontal-data platforms by consolidating different types of cloud workloads from analytical, transactional, structured/unstructured, ETL, AI/ML, etc. into a single platform.
- This year, the focus for both companies has been less on supporting new data types, workloads, and formats and more on building out different approaches to operationalize and extract value from the large amounts of proprietary data that already live inside Databricks’ and Snowflake’s cloud-data platforms.
- Databricks, the cloud ML-platform: Databricks’ product announcements highlighted the modularity of its platform. These included Unity Catalog, a data catalog which serves as a single layer . While Databricks already has many of the data engineering (ex. Delta Live Tables and Autoloader for ETL pipelines), science (ex. MLflow) and analytics (ex. Databricks SQL and Photon SQL runtime) modules built on top of its data lake, last week the company announced Lakehouse AI, its Generative AI module. This included the company’s own vector-search index, feature store and serving layer, and model repository, populated with Dolly, Mosaic MPT and other open-source models, and a serving and monitoring layer. Databrick’s expanding product breadth showcases a clear strategy to build workload-specific modules on top of its core data platform (delta lake + unity catalog) and expand to other personas.
- Snowflake, the full stack data cloud: Snowflake, on the other hand, continues to straddle between analytics and operational use cases with Unistore. The company’s closed-garden approach has made it difficult for the company to expand to new personas beyond data analysts and, as a result, Snowflake has focused its efforts and product releases on building out high-level applications for business users. This includes new product releases around Document AI, Neeva for enterprise search, etc.
Open vs. closed:
- At a high level, both events showcased each company’s respective strengths, and the reality that both are coming at AI from opposite ends of the technology spectrum—positions that may determine who ultimately emerges with a bigger share of the giant AI market as virtually every company starts to leverage AI technology.
- Snowflake has its roots as a data warehousing/structured BI analytics provider, offering a closed platform that caters more to data analysts. Databricks, on the other hand, has open-source roots and appeals to data scientists and data engineers. Databricks started off offering “data lakes”—centralized repositories for storing structured and unstructured data—which naturally hold more of the unstructured data necessary to train today’s AI/ML models.
- In this way, we think Snowflake’s journey to AI workloads is a longer one than Databricks’, putting Databricks in a better pole position to ultimately win this race. Databricks’ initial bets allow the company to, potentially, own the full ML lifecycle, including model training; model fine-tuning; model delivery; prompt engineering; and vector engineering, which not only unlocks the competitive advantages of injecting proprietary first-party, enterprise data into the AI workflow, but also arms the company with a broad offering that could allow it to benefit regardless of how the AI market evolves. Snowflake is much more beholden to third-party models today.
Battle for the developer:
- While both companies started with a focus on data personas (analysts, engineers, scientists), both companies are now expanding further up the stack to capture developers as well as non-technical, but highly analytical, business users by delivering higher levels of abstraction and more-advanced analytical features to reduce the time and effort required to get to insights.
- The next phase of growth for these data platforms is predicated on winning the mindshare of the developers, both AI developers (Databricks Lakehouse AI) and application developers (Snowflake Unistore) alike.
- Platform functionality needs to expand beyond allowing developers to simply build and train a model; it’s also critical that developers have the tooling required to easily embed a model into an application to enable end-user consumption. We see the recent acquisitions of Mosaic by Databricks and Streamlit by Snowflake as examples of this.
For now, Databrick’s and Snowflake’s businesses remain somewhat complementary—many organizations run both Snowflake and Databricks—and, we think, will likely stay that way for a while. But the two user conferences made clear that both have the same goal: becoming the pre-eminent platform to turn help every company into an AI company. We are bullish on Databricks’ plan, through its Lakehouse AI product, to build end-to-end infrastructure to help companies convert data into their own ML models, and to serve as the hub into which for people can integrate critical data when they want to build ML models with data they’re already storing inside Databricks.
Indeed, we feel AI/ML models are becoming more commoditized as the cost curve to train and run models continues to come down; companies like OpenAI offer products like SaaS offerings; and open-source makes high-quality models more accessible. Companies’ proprietary data may be their best AI “moat” to protect them from competitive threats. In today’s world, data is the core corporate asset, and it’s up to individual organizations to monetize and commercialize it through the new wave of tools companies like Databricks and Snowflake are developing. We’re excited to see this race continue!
The information contained above is based solely on the opinions of Dharmesh Thakker, Danel Dayan, Jason Mendel, Sudheendra Chilappagari and Patrick Hsu. It is material provided for informational purposes, and it is not, and may not be relied on in any manner as, legal, tax or investment advice or as an offer to sell or a solicitation of an offer to buy an interest in any fund or investment vehicle managed by Battery Ventures or any other Battery entity.
The information and data are as of the publication date unless otherwise noted.
Content obtained from third-party sources, although believed to be reliable, has not been independently verified as to its accuracy or completeness and cannot be guaranteed. Battery Ventures has no obligation to update, modify or amend the content of this post nor notify its readers in the event that any information, opinion, projection, forecast or estimate included, changes or subsequently becomes inaccurate.
*Denotes a Battery portfolio company. For a full list of all investments and exits, please click here.
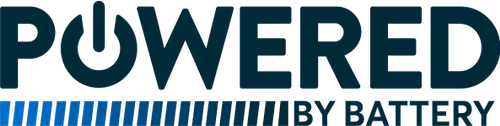
A monthly newsletter to share new ideas, insights and introductions to help entrepreneurs grow their businesses.