Predicting the weather—especially in this age of climate change—is big business. Today, the market for weather-forecasting services is $10 billion in the U.S., where an estimated one-third of the economy is exposed to weather and climate. Industries ranging from agriculture to commerce to the military are key consumers of weather-forecasting technology, most of which is based on information provided by large, national weather services.
But today, exciting new technologies are emerging that promise to improve the accuracy, timeliness and cost of weather forecasting, potentially at a lower cost. These include new weather data sources; forecasting approaches grounded in machine learning; and new applications for end users. We’re already seeing startups emerge to leverage many of these new technologies.
In our view, these innovations could not come soon enough. In the U.S. alone, natural disasters costing over $1 billion have increased in frequency from 13.1 per year in the 2010s to over 20 per year over the last 5 years. We see broad consensus among climatologists and insurers that the frequency, severity and impact of these events will increase in the years to come, making next-generation forecasting technologies even more critical.
The highest value opportunities we have identified through our market research include disaster mitigation and preparation for state and local governments; mission planning for defense; route planning and ground operations for airlines; route optimization for logistics companies; energy-demand forecasting for utilities; and conditions-based marketing for the travel and leisure industry.
The science of weather—a look back
Taking a step back, it’s clear that atmospheric science has made enormous strides since the first “numerical weather prediction” (NWP) models were conceived in the 1950s. Though weather is stochastic to the core, the well-understood physical laws of hydrodynamics long have been applied to predict the weather with useful near-to-midterm accuracy. Instantiating these laws in predictive models requires simulating the earth’s atmosphere as a three-dimensional grid of cells exchanging energy and moisture from observed starting conditions. This requires some of the most complex and demanding computational workloads in existence.
Consequently, the forecasts we all rely upon today are generally provided by large national weather services—most importantly, the National Oceanic and Atmospheric Administration (NOAA) and National Weather Service (NWS) in North America and the European Centre for Medium-Range Weather Forecasts (ECMWF) in Europe, supplemented by several country-specific models such as the Unified Model (UM) in the U.K. and the ICON Model in Germany.
Overview of NWP from Google Research.
But there are new possibilities to innovate on this foundation, including through the same forces spurring innovation in so many other industries: novel sources of data complementing large data sets for training, declining costs of computation and machine-learning (ML) techniques capable of discerning patterns beyond human inference.
In the case of weather, the theoretical promise of ML-weather prediction is to “abstract away” a considerable portion of the traditional, physics-based modeling in order to generate accurate predictions with far lower computational demand—i.e., to do it both faster and cheaper. Think of this as analogous to the way LLMs can generate highly useful text output without (discernibly) learning the rules of language semantics and syntax.
A figure from Forecasting Global Weather with Graph Neural Networks (Ryan Keisler, PhD) showing a Coder → Processor → Decoder approach for weather prediction using neural networks.
Early indications for new, ML-based forecasting approaches are encouraging. DeepMind reports its GraphCast model produces more accurate predictions than the ECMWF’s leading HRES model on over 90% of 1,380 test variables and forecast lead times, and Nvidia claims its FourCastNet model can compute forecasts orders of magnitude faster (and thus cheaper) than ECMWF models with comparable or superior accuracy.
Newer vendors like Atmo, Zeus AI, and WindBorne Systems similarly claim their ML models offer greater accuracy and granularity than incumbent NWP models. Other companies are providing ML weather forecasting that is fine-tuned to serve specific use cases, such as Jua (for energy trading) and Excarta (for insurance and solar).
While there is near-consensus among industry and academic experts that deep-learning approaches will play a role in improving forecasts, questions remain. Explainability, the unclear incorporation of physical constraints, the potential for hallucination and the ability to account for rare but extreme weather events are all hurdles that ML models still have to clear.
Most importantly, ML models have not yet been rigorously tested over a meaningful time period across mission-critical use cases. The prevailing view among experts we surveyed is that the likely end-state will be hybrid approaches that combine deep learning with hard-earned insights of physics-based NWP.
“The progress of the pure machine-learning models has been quite astonishing during [1H 2023] and many scientists in the field have been taken by surprise regarding the quality of predictions.”
– Peter Deuben, head of Earth system modeling at ECMWF
“The results of [ML-based forecasting systems] are amazing.”
– Hendrik Tolman, National Weather Services senior advisor for modeling
DeepMind’s Graphcast model shows meaningfully less error than HRES, the leading ECMWF model.
As ML models grow in sophistication, a key challenge will be data assimilation—i.e., the incorporation of novel data sources that are structured differently in time and granularity. Companies such as Spire, DTN and Tomorrow.io collect advanced weather data through weather ground stations, satellites (for imagery, radio occultation, etc.), sensors and space-based radar, which could potentially expand coverage of the atmosphere to large portions of the world that are poorly surveyed today.
WindBorne, for example, collects atmospheric data from its weather balloons, which can navigate over oceans and rainforests to obtain hard-to-reach data. This type of new data holds the promise of improving the accuracy of models, though the ability to fully incorporate novel data appears to be still in its infancy.
Overview of WindBorne’s innovative global sounding balloons (GSBs).
Beyond forecasts and data, another key area for value creation in weather is business applications that translate weather forecasts into actionable insights, such as planning the most efficient flight or trucking route, deciding whether or not to cancel an outdoor concert due to thunderstorms or launching a weather-driven ad campaign.
Consumer-facing weather applications represent another intriguing opportunity: Weather forecasts are checked over 300 billion times per year in the U.S., and close to nine out of 10 adult Americans obtain weather forecasts three or more times each day.
Overall, we are excited about the promise of ML in weather forecasting, powered by new sources of high-resolution data and translated into useful business and consumer applications.
Not only could these improvements create significant fundamental value for thousands of public-sector, military and commercial customers, but they could potentially unlock new use cases and expand coverage to constituencies underserved by governmental incumbents.
If you are building or investing in ML for weather forecasting, we would love to hear from you.
The information contained herein is based solely on the opinions of Marcus Ryu and Adam Piasecki and nothing should be construed as investment advice. This material is provided for informational purposes, and it is not, and may not be relied on in any manner as, legal, tax or investment advice or as an offer to sell or a solicitation of an offer to buy an interest in any fund or investment vehicle managed by Battery Ventures or any other Battery entity.
The information and data are as of the publication date unless otherwise noted. Content obtained from third-party sources, although believed to be reliable, has not been independently verified as to its accuracy or completeness and cannot be guaranteed. Battery Ventures has no obligation to update, modify or amend the content of this post nor notify its readers in the event that any information, opinion, projection, forecast or estimate included, changes or subsequently becomes inaccurate.
The information above may contain projections or other forward-looking statements regarding future events or expectations. Predictions, opinions and other information discussed in this video are subject to change continually and without notice of any kind and may no longer be true after the date indicated. Battery Ventures assumes no duty to and does not undertake to update forward-looking statements.
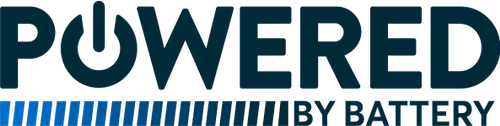
A monthly newsletter to share new ideas, insights and introductions to help entrepreneurs grow their businesses.