As a data scientist, there’s a good chance that you’ve experienced the frustration that stems from spending a seemingly endless number of hours curating and preparing the clean and representative dataset that’s needed to power your machine learning (ML) model. We’re here to shed light on your frustration and tell you that you’re not alone—and that there’s new technology available to help.
At its core, ML is a big and messy data problem, and models—which are deployed across industries to automate core business tasks and increase efficiency—require massive amounts of data before they can be reliably used in production. To put it simply, a model can only be as good as the data that it’s trained on, and poor-quality model predictions are often caused by erroneous or poor quality data. Data intelligence—or the ability to holistically understand and improve the health of the data that’s powering the model—is one of the most critical, yet underappreciated, considerations for any organization that’s looking to successfully reap the benefits of ML.
Underpinning the intense focus on data intelligence is the tectonic shift from model-centric to data-centric artificial intelligence (AI). Recent advancements, including widely available, off-the-shelf pre-trained models and powerful new ML frameworks, have democratized access to complex, high-performance models and shifted the focus away from the model and towards the data. Under the data-centric AI paradigm, the best way to improve the model’s health and performance is by improving the quality of the underlying data that’s flowing through the model.
As AI adoption continues to increase, so too does the importance of data intelligence, without which it’s next to impossible to understand and inspect large sets of ML data. Determining the mix of optimal data on which to label and train a model—plus continuously uncovering and fixing data errors—has become a messy and time-consuming process. Errors in the data can stem from a number of issues, including missing or insufficient data, too much data, mislabeled data and stale data. And data-quality issues, while challenging to spot with the naked eye, can have a catastrophic impact on the model’s performance.
It’s therefore unsurprising that ML developers are spending so much time optimizing the data that’s powering their models; however, much of that workflow is ad-hoc and manual today, and ML developers lack a standard set of tools to intelligently understand and manage data at scale and proactively improve the performance of models.
Enter Galileo*
Galileo was purpose-built to solve ML’s messy data problem and serves as a layer of intelligence to help data scientists manage data throughout the ML lifecycle. Using Galileo’s technology, data scientists can easily visualize the data that’s flowing through their models, curate the right data for model training, track and collaborate across datasets, and identify and debug costly ML data errors, such as missing data or labeling errors. This leads to less time and money spent on data preparation and, most importantly, better model performance through better quality data. Galileo’s product is already being used in production by a handful of early adopters at Fortune 500 companies and startups across multiple industries.
The company was founded in 2021 by Vikram Chatterji, Atindriyo Sanyal and Yash Sheth, a team of ML experts who experienced AI’s messy data problem first-hand while building and deploying models at some of the world’s largest AI-first companies. Vikram and Yash previously worked on large-scale AI projects at Google, and Atindriyo previously helped build out Uber’s Michelangelo platform and was an early member of the Siri team at Apple. Through their prior experiences, the Galileo team has developed a wealth of knowledge and first-hand principles, which they’re using to solve one of ML’s most complex and pressing challenges.
We have been fortunate to partner with other companies across the AI / ML workflow: Databricks*, Arize*, Dataiku* and Paperspace*. We’re excited to work with Galileo as the company brings data intelligence to ML. We look forward to this next chapter of growth ahead.
This material is provided for informational purposes, and it is not, and may not be relied on in any manner as, legal, tax or investment advice or as an offer to sell or a solicitation of an offer to buy an interest in any fund or investment vehicle managed by Battery Ventures or any other Battery entity.
*Denotes a past or present Battery portfolio company. For a full list of all Battery Ventures investments, please click here. No assumptions should be made that any investments identified above were or will be profitable. It should not be assumed that recommendations in the future will be profitable or equal the performance of the companies identified above.
The information and data are as of the publication date unless otherwise noted.
Content obtained from third-party sources, although believed to be reliable, has not been independently verified as to its accuracy or completeness and cannot be guaranteed. Battery Ventures has no obligation to update, modify or amend the content of this post nor notify its readers in the event that any information, opinion, projection, forecast or estimate included, changes or subsequently becomes inaccurate.
The information above may contain projections or other forward-looking statements regarding future events or expectations. Predictions, opinions and other information discussed in this video are subject to change continually and without notice of any kind and may no longer be true after the date indicated. Battery Ventures assumes no duty to and does not undertake to update forward-looking statements.
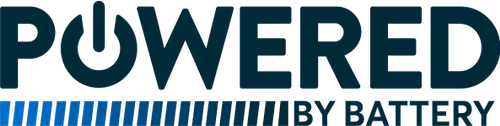
A monthly newsletter to share new ideas, insights and introductions to help entrepreneurs grow their businesses.