Since generative artificial intelligence (gen AI) became a household phrase in the last year, enterprise technology leaders have experienced incredible pressure to deploy the technology, between a groundswell of user requests and top-down mandates from boards.
However, actual enterprise implementation of AI is proving to be more complicated, something that could adversely impact the fortunes of software companies selling into the enterprise, at least in the near term.
Specifically, many organizations considering AI implementations are concerned about the potential loss of corporate data, a risk that weighs heavily on the minds of buyers and their legal counterparts, for example. On the vendor side, many software startups are scrambling to build gen AI products and workflows to enable new user experiences. But software companies cannot take a ‘build it and they will come’ approach but indeed need to satisfy AI-hesitant buyers – or worse, they may damage their long-term growth.
From our conversations with technology and risk-management executives, we have identified many of the primary concerns that enterprises are addressing in negotiations with new software sellers:
- Employee training: As workflows change to incorporate AI, many executives understand that this will require educating their employees on the associated risks, which can create a significant administrative burden.
- Risk assessment surveys and accreditation: Since gen AI is still an emerging technology, there are no industry-standard criteria for its implementation. Until this stabilizes, sellers will get a broad range of new questions in the vendor assessment process which will require attention from sales, product and other teams.
- Non-standard contractual agreements, such as indemnification: Since many AI products are built on third-party tools, sellers are seeing new contract clauses that can expose them to new risks.
- Zero-data retention: For security and data privacy reasons, some enterprises are requiring that their data not be retained for training or other uses.
- Tokenization of data: Often associated with retrieval-augmented generation (RAG), as well as data masking and redaction, tokenizing or obfuscating data into a model can limit its ability to perform iterative reasoning.
- Blocking services: Many enterprises’ knee-jerk reaction is to block large-language model (LLM) services such as ChatGPT, though many approved applications have embedded these LLMs into their product.
- Abstraction of underlying services: Potentially the most interesting (and disruptive) is the concept of abstracting underlying applications via an enterprise-built bot-of-bots.
We saw many of these challenges and anxieties from buyers with the emergence of SaaS years ago. Thankfully, over time, as tech, legal and compliance organizations gained more of an understanding of true risk associated with SaaS and cloud products, those concerns lessened, and the sales process became easier. That process took years, as will likely be the case for AI implementation.
Impacts on startups selling into the enterprise
A patchwork approach to AI implementation, through which cautious enterprise buyers exercise strict oversight over different tools to meet the individual needs of different parts of the organization, can hinder productivity, efficiency and strategy in myriad ways:
- Uncertainty and delays in sales cycles: Inconsistent risk assessments can introduce uncertainty and delays in a startup’s sales cycles. Potential customers may request multiple assessments, leading to prolonged negotiations and increased scrutiny of the product. This uncertainty can deter customers from adopting new products and slow the sales process.
- Increased costs: Working with multiple risk-assessment services providers can be expensive. Sellers need to pay for individual assessments, manage vendor relationships and allocate internal resources to coordinate the process. These costs can strain budgets and limit the resources available for product innovation.
- Splitting the roadmap: In prior technological shifts, such as the transition to the cloud, legacy software providers were forced by many of their largest customers to have a dual-product strategy. Since the product and engineering team has finite resources to maintain the infrastructure and code base, many software companies will make the decision to fire customers that will hold them back.
The fragmented risk landscape and resulting patchwork approach to AI implementation could, we fear, foster an inefficient enterprise environment in which both enterprises and software vendors manage through risk calculations. An industry-standard approach – alongside product and service standards – would simplify decision-making, improve risk-mitigation strategies, accelerate time-to-value cycles and ultimately, drive greater innovation.
Rethinking use of software
There is also an emerging shift greater than legal and product changes. The most successful B2B software companies have built products that engage users in the product and charge based on increased productivity. Many of these revenue models are based on the number of employees with a login. This calculus could change when the product is disintermediated by a higher-level bot.
An example from one major enterprise is a home-built internal gen AI bot that orchestrates across multiple apps and services for employees. For example, to request vacation time off, an employee will simply make the request in natural language to the internal bot. The bot will tee up the bots across a web of apps such as Workday, Office 365, ADP and others, to schedule time off and grant approval, creating efficiencies that track exactly to internal rules and guidelines.
We have seen industry specific companies, such as online travel aggregators (OTAs), abstract the customer away from the company providing the service such as hotels. The travel industry spent years re-building consumer loyalty.
In this bot-over-bot world, both enterprises and software startups will need to navigate what it means to build differentiated product experiences and business models that command the premium required to fund future innovation.
The information contained herein is based solely on the opinion of Scott Goering and Evan Witte and nothing should be construed as investment advice. This material is provided for informational purposes, and it is not, and may not be relied on in any manner as, legal, tax or investment advice or as an offer to sell or a solicitation of an offer to buy an interest in any fund or investment vehicle managed by Battery Ventures or any other Battery entity. The views expressed here are solely those of the author.
The information above may contain projections or other forward-looking statements regarding future events or expectations. Predictions, opinions and other information discussed in this publication are subject to change continually and without notice of any kind and may no longer be true after the date indicated. Battery Ventures assumes no duty to and does not undertake to update forward-looking statements.
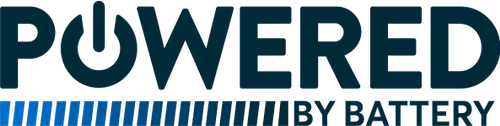
A monthly newsletter to share new ideas, insights and introductions to help entrepreneurs grow their businesses.